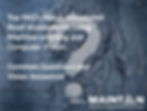
Understanding Automated Road Assessments: What You Need to Know
As our transportation networks continue to expand, road maintenance becomes an increasingly complex and costly endeavour. Thankfully, advances in technology have made it easier to gather and analyse data about road conditions, allowing for more efficient and effective maintenance strategies.
One of the most exciting developments in this area is the use of automated road assessments using machine learning and computer vision. This cutting-edge technology can help to identify issues with road surfaces, such as potholes, cracking and deterioration, with incredible speed and consistency. However, like any proposed change in approach through adopting innovative solutions, there are there are numerous questions that are raised. In this article, we will explore some of the most frequently asked questions and comments we receive about automated road assessments, and provide you with the facts you need to make informed decisions about this technology.
When working with a limited asset maintenance budget, the goal is to maximise the value of each dollar. This is what we aim to do. Good Roads Cost Less! Let's Save the Good Roads.
Where could AI-based road inspections help you? Explore the potential of AI in road maintenance with our evaluation tool; a data-driven approach to understanding and optimising your infrastructure strategies. Try it here.
Top Frequently Asked Questions (FAQ) Regarding Automated Road Assessments / Automated Highway Assessments using AI
Below we have considered the Top FAQ (Top questions or statements) we have been asked about Automated Road Assessments and Automated Highway Assessments:
Question 01
What is automated road assessment technology using machine learning and computer vision and how does it work in achieving better roads?
Answer
Automated road assessment technology, as offered by Maintain-AI, uses computer vision and machine learning algorithms to analyse data collected from mobile phone camera sensors mounted on vehicle windscreens that are driven along roads to automatically detect road defects.
Because of the ease of data collection, end users collect their own data because it is simple. This technology and process can detect various characteristics of the road surface, such as rutting, cracks, potholes, graffiti and other deterioration. The data collected is then analysed to provide a detailed health assessment of the road condition, including identification of any issues or hazards that require attention. This technology allows for faster and more accurate road assessments, which helps to improve road maintenance, make more informed decisions and reduce costs associated with traditional inspection approaches. As the process is very cost-effective and efficient, Maintain-AI enables you to examine your whole road network more regularly for less expense and time, ensuring you know the current health status of your pavement network on a consistent basis. As a result, this lowers the overall lifecycle cost of your roads when you adopt the philosophy of keeping the good roads good.
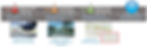
Question 02
What types of data can be collected through automated road assessments, how can this data be integrated into existing road asset management systems and can the technology be customised to meet the unique needs of different road networks and regions?
Answer
Automated road assessments using machine learning and computer vision can collect a range of data regarding road surface conditions, including information about potholes, cracks, other surface defects and deterioration. This data is analysed using machine learning models to identify and classify different types of defects and can be tabulated to prioritise repairs based on their severity and density. The data collected through automated road assessments can be integrated into existing road asset management systems, providing a comprehensive overview of the condition of the road network and informing decisions about maintenance and repairs. The technology can also be customised to meet the unique needs of different road networks and regions by adjusting the type of data collected, the frequency of assessments and the level of data analysis required. This flexibility can help road authorities and maintenance personnel to develop targeted maintenance strategies that are tailored to the specific needs of their road network, leading to more efficient and effective road maintenance operations.
Question 03
How does the cost of implementing automated road assessments compare to traditional inspection methods?
Answer
The cost of implementing automated road assessments can vary depending on a range of factors, including the size and complexity of the road network and the level of data analysis required, however, it can be significantly more cost effective than traditional approaches thus allowing for more frequent inspections which will lead to improved road maintenance and better roads for end users. Traditional inspection methods such as manual inspections or laser scanning vehicles require significant resources in terms of personnel, time and equipment. With automated road assessments, data can be collected at a faster rate and with higher consistency, leading to more targeted maintenance strategies that can reduce costs associated with road repairs and replacements. Additionally, automated assessments can provide more objective data that can inform decisions about the allocation of resources for road maintenance, ensuring that investments are targeted to the most critical areas.
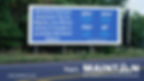
Question 04
As road owners and infrastructure managers increasingly seek more efficient and advanced asset management frameworks to optimise their resources and deliver better outcomes, a pertinent question arises: How can automated AI road assessments, like Maintain-AI, transform ISO 55001-driven pavement management systems?
Answer
Automated AI pavement assessments, as supported with Maintain-AI's solution, introduces a transformative step change in ISO 55001-driven pavement management systems—a change that's urgently needed in today's dynamic infrastructure landscape.
While ISO 55001 provides a robust framework for asset management, integrating AI-driven automated road inspection solutions can significantly enhance the efficiency, objectivity and proactiveness of these systems. Automated assessments, powered by AI, offer rapid, consistent and frequent data collection. This not only allows for more regular inspections but also facilitates significantly better data-driven decision-making. The result is a more targeted and effective maintenance strategy, ensuring that resources (cost, time and materials) are allocated where they are most needed, ultimately leading to improved road conditions, safer roads, more sustainable road asset lifecycles, extended asset lifespans and cost savings.
Viewpoint 05
Traditional techniques such as laser scanning vehicles provide a higher level of accuracy and precision in identifying road surface defects and damage.
Response
While laser scanning vehicles (e.g. ROMDAS and ARAN) may provide a high level of accuracy and precision in road condition surveys, they are also expensive, time-consuming to operate and are thus utilised too infrequently to improve maintenance practices. Automated road survey systems using machine learning and computer vision can be conducted more quickly and efficiently, allowing for more frequent inspections and more proactive maintenance strategies. Additionally, with advances in artificial intelligence technology, automated road surface inspections are becoming increasingly accurate and can provide comparable results to traditional techniques in certain conditions.
It's important to clarify that we are not advocating for the complete replacement of laser scanning vehicles like ROMDAS and ARAN. Instead, we see immense value in integrating automated road assessment using AI as part of a comprehensive road maintenance strategy. The agility and efficiency of AI-driven systems enable swift identification of road changes. When used in tandem with laser scanning vehicles, AI can enhance the frequency and timing of scans, optimising their deployment. This hybrid approach ensures a more adaptive, timely, and cost-effective road maintenance strategy, harnessing the strengths of both methodologies.
Viewpoint 06
Manual inspections allow for a more detailed analysis of road assets, including identifying less obvious issues that may be missed by automated inspections.
Response
While manual inspections can provide a detailed analysis of road assets, they are also prone to human error and are typically subjective. Additionally, due to resource constraints, it is not possible to manually inspect all facets of a road network frequently hence manual inspections are sometimes limited to a representative sample of network to provide a general indication of road health. Automated road inspections using machine learning and computer vision can provide objective and consistent assessments very efficiently, leading to more effective asset management strategies. These inspections can also assess many more attributes across the entire network during the same data collection survey. Additionally, automated inspections mitigate issues related to safety, protecting inspectors from potentially hazardous site conditions.

Viewpoint 07
Automated inspections using machine learning and computer vision lack the ability to make subjective judgments and interpretations of road conditions, which can lead to inaccurate assessments.
Response
While automated inspections may not be able to make subjective judgments, they are not prone to the same biases and inconsistencies that can occur with human inspections. Machine learning algorithms can be trained to identify specific types of road defects, such as pavement deterioration, with a high level of consistency, providing very reliable data for road authorities to make more effective maintenance decisions.
Viewpoint 08
Traditional techniques such as manual inspections and laser scanning vehicles provide a human element that can understand and address the unique characteristics of a specific road network.
Response
While the human element is important in road maintenance and asset management, automated inspections using machine learning and computer vision can provide a more consistent and objective assessment of road conditions. By removing the potential for human error and biases, automated inspections can provide more frequent and reliable data that can be used to inform maintenance strategies. Automated road survey methodology can be tailored to specific road networks by adjusting the machine learning algorithms used.
Viewpoint 09
Automated road inspections may not be able to detect certain types of road surface damage.
Response
While there may be some types of road surface damage that automated inspections cannot detect readily, advances in machine learning and computer vision technology are making automated road condition surveys increasingly accurate and reliable. In addition, automated road surface inspection systems can be used in conjunction with other inspection approaches to provide a more comprehensive and frequent analysis of road conditions or be used as a quick filtering tool to better understand the actual condition state of a road network to support prioritising which pavements may require more detailed inspection. Notwithstanding, more frequent inspections through automated machine learning and computer vision technology provides more data points to support making more informed decisions.
Viewpoint 10
Manual inspections and laser scanning vehicles can provide a more comprehensive analysis of road assets, including identifying changes in the surrounding environment and potential hazards.
Response
Automated road surface inspections using machine learning and computer vision can not only provide comprehensive analysis of road assets, but also continually improve their performance through ongoing data collection and algorithm updates. Advanced algorithms can be trained to identify changes in the surrounding environment and potential hazards, which can be addressed proactively. Furthermore, with continuous advancements in computer vision and machine learning, automated inspections are becoming increasingly accurate and efficient and offer a cost-effective and reliable methodology for road authorities, reducing the impact of human errors and optimising maintenance resource activities.

Viewpoint 11
Automated inspections are limited by the quality of the data that they are able to collect, which can be affected by various factors such as lighting and camera quality.
Response
While it is true that the quality of data collected by automated inspections can be affected by certain factors, continuous advancements in camera sensor technology and image processing techniques help mitigate these issues. By combining data from multiple sensors and using advanced algorithms, automated road surface inspections can enhance the overall quality and reliability of the collected data, leading to more accurate and detailed analysis of road conditions. In addition, automated road survey systems can gather data more efficiently and broadly, making them an essential solution for road maintenance and asset management strategies.
Viewpoint 12
Traditional techniques such as manual inspections and laser scanning vehicles are able to adapt to changing conditions, while automated inspections are limited by their pre-programmed algorithms.
Response
Adaptive machine learning algorithms and advanced camera sensors enable automated road surface inspections to adapt to changing conditions, providing an advantage over traditional techniques. These systems can learn from new data that is added and adjust their analysis and detection capabilities accordingly once trained, allowing for near real-time road inspection outcomes that can support enabling more timely maintenance and repairs. This technology can greatly improve road asset management and reduce costs by detecting deterioration early and prioritising resources efficiently.
Viewpoint 13
Automated inspections may not be able to differentiate between superficial and more serious road surface damages, which can lead to a waste of resources in addressing minor issues.
Response
Advanced machine learning algorithms and computer vision techniques can be trained to differentiate between superficial and more serious road surface damage. This enables automated road surface inspections to identify areas of criticality to prioritise maintenance tasks and allocate resources more effectively, ultimately supporting the better use of resources in addressing various issues. Automated road data collection ensures accurate and consistent information, allowing road authorities to make effective decisions based on objective surface condition assessments.
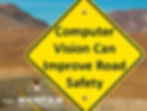
Viewpoint 14
Manual inspections and laser scanning vehicles provide a more interactive and engaging approach to road maintenance inspections and road asset management, which can encourage greater collaboration and communication among stakeholders.
Response
Automated inspections can complement traditional techniques and improve collaboration and communication among stakeholders by providing a consistent, objective and data-driven assessment of road conditions. The data gathered by automated road survey systems can be easily shared among stakeholders, facilitating better decision-making and more effective road maintenance and asset management strategies. This can lead to improved safety and performance of the road network, ensuring a well-maintained road network for economic and social development.
Viewpoint 15
Traditional techniques such as manual inspections and laser scanning vehicles offer the opportunity for immediate and real-time feedback, allowing for timely maintenance and repairs.
Response
Automated road surface inspections can equally provide near real-time feedback and immediate analysis, allowing for timely maintenance and repairs. By using advanced camera sensor technology provided in today's more modern mobile phones and artificial intelligence, these systems can rapidly process and analyse data, ensuring that road authorities receive up-to-date information on road conditions. This enables them to readily identify and prioritise maintenance tasks and allocate resources more effectively, leading to better overall road asset management and improved safety for road users.
Viewpoint 16
Automated inspections may struggle to accurately assess the condition of certain types of road surfaces, such as those made of unconventional materials.
Response
While automated inspections may initially struggle to accurately assess certain types of road surfaces, continuous advancements in machine learning algorithms and sensor technology enable them to adapt and improve over time. By incorporating additional data sources and refining the algorithms, automated road surface inspections can become increasingly proficient in assessing various road surface types, including those made of unconventional materials. This adaptability enhances their effectiveness as a solution for road maintenance inspections and road asset management strategies. In such scenarios. Maintain-AI can work collectively with you to determine how best to support such use cases.

Viewpoint 17
Road inspections require millimetre precision to adequately understand pavement conditions and automated road surface inspections using machine learning and computer vision may not provide this level of precision, rendering this approach ineffective in road maintenance inspections and road asset management strategies.
Response
While the concern for millimetre accuracy in crack detection is understandable, it is important to consider the broader impact of inspection frequency on road networks. Automated road surface inspections using machine learning and computer vision enable more frequent inspections, increasing the likelihood of identifying issues that can improve maintenance and overall road network health. Furthermore, automated inspections can determine defect severity and the precision of these inspections is largely dependent on factors such as photo quality and lighting conditions, which can be optimised to achieve higher accuracy. In this context, the benefits of more frequent and adaptable automated inspections outweigh the need for absolute precision in every case.
Viewpoint 18
Manual inspections and laser scanning vehicles provide an opportunity for workers to observe and identify underlying issues or potential hazards and detect road defects that may not be apparent through automated inspections.
Response
Automated road surface inspections can provide valuable insights into potential hazards and underlying issues that may not be apparent through manual inspections. By leveraging machine learning algorithms and advanced camera sensor technology, these systems can detect subtle patterns and anomalies in the road surface, allowing for the identification of issues that might have been overlooked by human inspectors. While an issue may be missed during a single survey, regardless of method, the reality that automated road surface inspections are more cost-effective and efficient than other traditional approaches supports the philosophy that more consistent inspections increase the likelihood that defects will be detectable in subsequent surveys. This ability to uncover hidden issues makes automated road surface inspections an essential component of road maintenance inspections and road asset management strategies.
Viewpoint 19
Automated Technologies Elevate Road Safety and Performance Through Consistent Detection of Road Surface Distress.
Response
Automated road assessment methods and technologies significantly enhance the detection of road surface distress, playing a pivotal role in maintaining and improving road quality and safety. By utilising advanced algorithms, automation and high-resolution imagery to assess road condition data, these systems analyse road images and provide a comprehensive and detailed analysis of a pavement surface and general road conditions, identifying various types of surface distress such as cracks, potholes and hazards with objective consistency. This capability allows for the early detection of potential hazards, facilitating prompt maintenance actions that prevent minor issues from escalating into serious problems.
Moreover, the integration of automatic road assessments into road and highway maintenance strategies enables a more dynamic approach to managing road performance. By continuously monitoring road conditions, these technologies offer invaluable insights into the lifecycle of road infrastructure, supporting the development of predictive maintenance schedules that will further optimise resource allocation and minimise disruptions to road users.
The deployment of automated technologies in road assessments not only elevates the standard of road safety but also ensures a sustainable approach to road asset management. By prioritising interventions based on the severity and urgency of detected distress, road authorities can extend the lifespan of road infrastructure, enhance user experience and ensure a safer journey for all. This strategic advantage underscores the critical role of automated road condition evaluation in the modernisation of road maintenance practices, aligning with the goals of improving road performance and safeguarding public safety.
Viewpoint 20
Automated road condition assessments lack the ability to provide recommendations for long-term road maintenance strategies, whereas traditional techniques can offer expert opinions based on their experience and observations.
Response
Automated road surface inspections, when integrated with data analytics and predictive modelling, can provide valuable insights for long-term road maintenance strategies. These systems can process large volumes of data and identify trends, allowing for more accurate predictions of future road conditions and the development of effective maintenance plans. This, along with the involvement of experienced road maintenance experts, and the fact that the data gathered is preserved and can be seen at any time through a web browser, can lead to a complete and data-driven approach to long-term road maintenance strategies.
Viewpoint 21
Traditional techniques such as manual road inspections and laser scanning vehicles can identify issues with road assets that are not immediately visible, such as the condition of underground utilities or road drainage systems.
Response
While some issues, like the condition of underground utilities or road drainage systems, may not be immediately visible to automated inspections, these systems can be integrated with other sensing technologies, such as ground-penetrating radar and LIDAR, to detect and analyse hidden issues. By combining data from multiple sources and leveraging advanced analytics, automated road surface inspections can provide a comprehensive assessment of road assets, including those that are not immediately visible. This combination of technologies results in a powerful and efficient tool for road maintenance inspections and road asset management strategies.

Viewpoint 22
The systematic collection of data using artificial intelligence is pivotal in advancing the approach to road condition assessments, contrasting with traditional methods that rely heavily on manual observation and periodic surveys. This modern strategy, facilitated by automated support systems, refines every aspect of road asset management.
Response
Technologies that support automated data acquisition enable a continuous flow of information, enhancing the consistency and breadth of road condition analysis. This approach not only streamlines the identification of issues but also fosters a more agile and responsive road maintenance framework. Coupled with advanced analytics, the data collected becomes a cornerstone for strategic planning, offering a path towards more sustainable and efficient road asset management practices.
Summary
Automated road assessments using machine learning and computer vision technology enable road authorities and road maintenance professionals to undertake faster, more consistent and significantly more cost effective road surface inspections across their entire pavement network.
This innovative technology approach can detect various road characteristics, such as rutting, cracks, potholes and deterioration and provide detailed assessments of road conditions to help improve road maintenance and reduce road maintenance costs.
The technology can be customised to meet the unique needs of different road networks and regions and integrated with existing road asset management systems to provide more comprehensive and detailed data for decision-making.
By detecting issues early through more frequent inspections, road authorities can take preventative action before they become more serious and expensive to repair. The data collected through automated road assessments can also help to improve road safety by identifying potential hazards and issues that could impact the safety of drivers and other road users.
Remember, good roads should cost less! Let's work together.
Let us know your thoughts?
Drive safely;
info@maintain-ai.com
About Maintain-AI:
Maintain-AI aspires to support Governments, other Road Asset owners and Industry professionals transform pavement and network assessments through AI-driven solutions. Founded on the principle that "Good Roads Should Cost Less", we harness the power of computer vision and machine learning to automate road surface inspections. Our state-of-the-art tools detect road defects and assess related infrastructure, enabling professionals to make data-driven decisions. By advocating for the optimal use of maintenance budgets, we emphasise that well-maintained roads are more cost-effective across a road's complete asset lifecycle. Our commitment is to support regular, objective network inspections, ensuring that every maintenance dollar is maximised. With Maintain-AI, infrastructure asset management is not only efficient but also offers a clear return on investment through maintenance savings. Join us in our mission to make roads better, safer, more sustainable and more cost-effective. All road users deserve it.
road inspection FAQ
digital road inspections
digital asset management
digital transformation